
Simulation Tutorial (Gaussian data)
Source:vignettes/Simulation_GaussianDat.Rmd
Simulation_GaussianDat.Rmd
In this tutorial, we’ll start by generating some simulated
spatio-temporal data, with the city of Bristol as our study region.
Next, we’ll walk you through the steps of running the Bayesian
Hierarchical Model (BHM) using the INLA-SPDE approach. Finally, we’ll
demonstrate the use the key functionality provided by the
fdmr
package.
Data simulation
First we simulate some Gaussian distributed data observed at 55
spatial locations in Bristol, UK and across 6 time points. We use the
fdmr::retrieve_tutorial_data
function and the
fdmr::load_tutorial_data
function to retrieve and load in
the geographical information for the spatial locations in Bristol from
the fdmr
example data store.
fdmr::retrieve_tutorial_data(dataset = "priors")
## The legacy packages maptools, rgdal, and rgeos, underpinning the sp package,
## which was just loaded, were retired in October 2023.
## Please refer to R-spatial evolution reports for details, especially
## https://r-spatial.org/r/2023/05/15/evolution4.html.
## It may be desirable to make the sf package available;
## package maintainers should consider adding sf to Suggests:.
##
## Tutorial data extracted to /home/runner/fdmr/tutorial_data/priors
sp_data <- fdmr::load_tutorial_data(dataset = "priors", filename = "spatial_dataBris.rds")
Then we simulate the Gaussian data that exhibit spatio-temporal
correlation. These simulated data are finally organized into a data
frame named simdf
, where each row corresponds to an
observation at a specific location and time. The variable
ID
is the spatial location identifier. Variable
time
denotes the time point. The variable datn
represents the simulated response data. The longitude and latitude
coordinates for each location are stored in the variables
LONG
and LAT
, respectively.
library(INLA)
## Loading required package: Matrix
## Loading required package: sp
## This is INLA_23.09.09 built 2023-09-09 13:43:09 UTC.
## - See www.r-inla.org/contact-us for how to get help.
n.time<-6
n <- 55
locs<- as.matrix(sp_data@data[,c("LONG","LAT")])
initial_range <-diff(range(locs[,1]))/3
max_edge<-initial_range /2
mesh <- fmesher::fm_mesh_2d(loc=locs,
max.edge = c(1,2)*max_edge,
offset=c(initial_range , initial_range ),
cutoff = max_edge/7
)
spde <- INLA::inla.spde2.pcmatern(
mesh = mesh,
prior.range = c(initial_range , 0.5),
prior.sigma = c(1, 0.01)
)
sigma0 <- 7
range0 <- 0.03
kappa0 <- sqrt(8 / 1) / range0
tau0 <- 1 / (sqrt(4 * pi) * kappa0 * sigma0)
inla.seed <- sample.int(n=1E4, size=n.time)
Q <- INLA::inla.spde.precision(spde, theta = c(log(tau0), log(kappa0)))
x.mat<-matrix(NA,ncol=n.time,nrow =mesh$n)
for (co in 1:ncol(x.mat)){
x.mat[,co]<-INLA::inla.qsample(n = 1, Q)
}
A <- INLA::inla.spde.make.A(mesh=mesh, loc=locs)
x.dat<-matrix(NA,ncol=n.time,nrow =n)
for (t in 1:ncol(x.dat)){
x.dat[,t] <- drop(A %*% x.mat[,t])
}
alpha<-0.9
sp.mat<-matrix(NA,ncol = n.time,nrow = n)
sp.mat[,1]<-x.dat[,1]
for (t in 2:n.time){
sp.mat[,t]<-alpha*sp.mat[,t-1]+x.dat[,t]
}
beta0 <- 0.5
sigma_e <- 0.1
lin.pred <- beta0 + sp.mat
y.mat <- lin.pred+matrix(rnorm(n*n.time,0,sigma_e),ncol=n.time)
simdf<-data.frame(ID=rep(1:n,each=n.time),
time = rep(c(1:n.time),n),
datn = as.numeric(t(y.mat)),
LONG = rep(locs[,1],each=n.time),
LAT = rep(locs[,2],each=n.time))
A histogram is created to visualize the distribution of the simulated Gaussian data.
hist(simdf$datn,main='', xlab='Data')
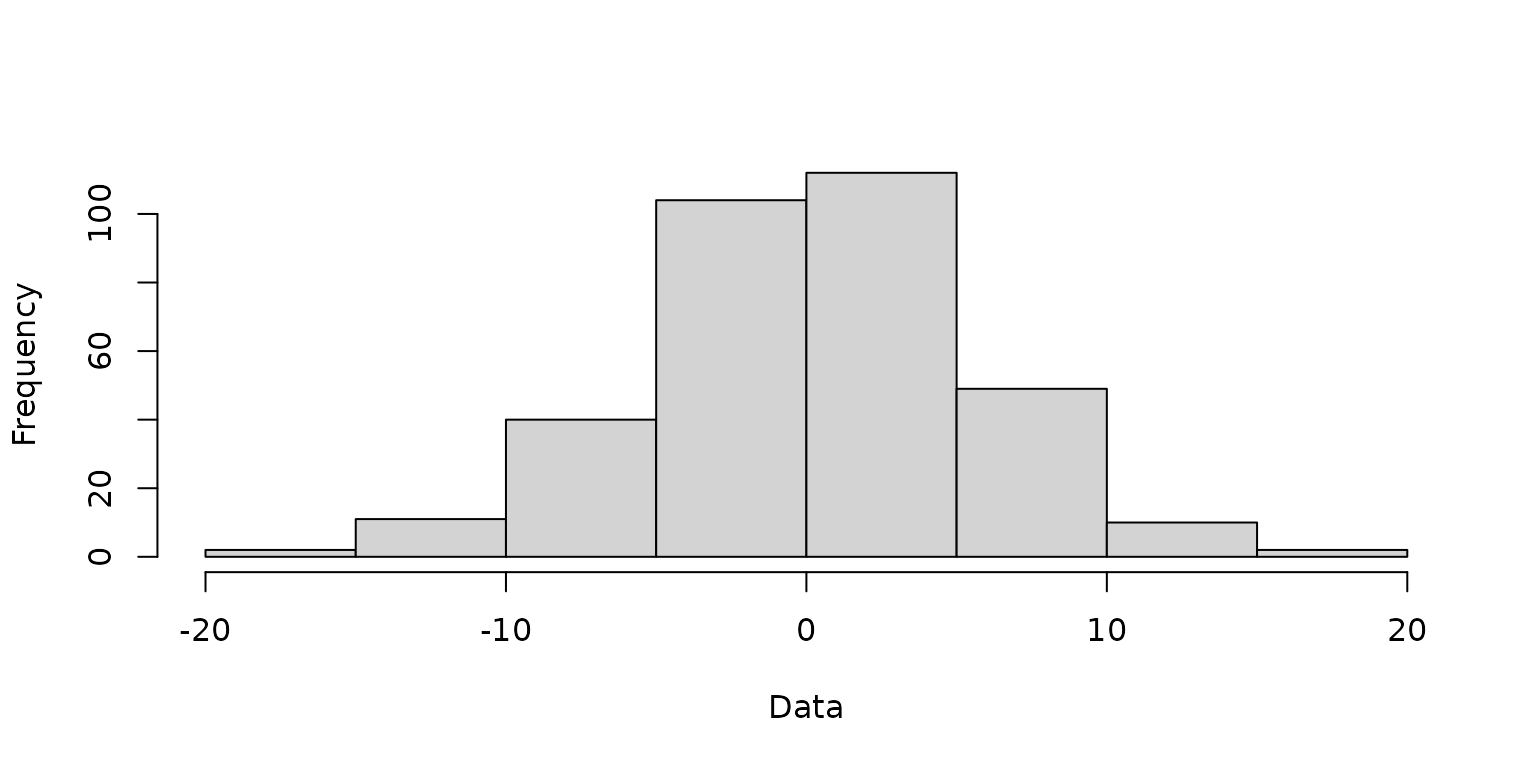
A histogram of the simulated Gaussian data.
We can also map the simulated data using the
fdmr::plot_map
function. For example, a map of the
simulated data at the first time point is given as
fdmr::plot_map(
polygon_data = sp_data,
domain = simdf[simdf$time==1,"datn"],
palette = "Reds",
legend_title = "Data",
add_scale_bar = TRUE,
polygon_fill_opacity = 1,
polygon_line_colour = "transparent"
)
A map of the simulated data in Bristol at the first time point.
Model fitting
With the simulation dataset now prepared, our next step is to implement a Bayesian spatio-temporal model using the INLA-SPDE approach.
Generate mesh, build the SPDE model, and set priors for the spatial parameters
locs <- unique(simdf[, c("LONG", "LAT")])
initial_range <-diff(range(locs[,1]))/3
max_edge<-initial_range /2
mesh <- fmesher::fm_mesh_2d(loc=locs,
max.edge = c(1,2)*max_edge,
offset=c(initial_range , initial_range ),
cutoff = max_edge/5
)
spde <- INLA::inla.spde2.pcmatern(
mesh = mesh,
prior.range = c(initial_range , 0.5),
prior.sigma = c(1, 0.01)
)
Define how the process evolves over time, set prior for the temporal parameter and define a temporal index
rhoprior <- base::list(theta = list(prior = 'pccor1', param = c(0, 0.9)))
group.index<-simdf$time
n_groups<-length(unique(simdf$time))
We will use the function bru()
of package
inlabru
to fit the model. bru
expects the
coordinates of the data, thus we transform simdf
data set
to a SpatialPointsDataFrame using the function
coordinates()
of the sp
package.
sp::coordinates(simdf)<- c("LONG","LAT")
Fit the model
Finally, we fit the spatio-temporal model using SPDE approach and
AR(1) process by calling the function bru()
of the package
inlabru
.
inlabru_model <- inlabru::bru(formula,
data = simdf,
family = "gaussian",
options = list(
verbose = TRUE
)
)
## Warning in add_mapper(component$main, label = component$label, lhoods = lh, : All covariate evaluations for 'Intercept' are NULL; an intercept component was likely intended.
## Implicit latent intercept component specification is deprecated since version 2.1.14.
## Use explicit notation '+ Intercept(1)' instead (or '+1' for '+ Intercept(1)').
## Warning in handle_problems(e_input): The input evaluation 'Intercept' for
## 'Intercept' failed. Perhaps the data object doesn't contain the needed
## variables? Falling back to '1'.
Results summary
After completing the model fitting process, you can examine the main
result summaries by typing summary(inlabru_model)
.
Additionally, you can check the parameter estimates for fixed effects,
random effects, and hyperparameters as follows.
model_summary<-summary(inlabru_model)
model_fixed <- inlabru_model$summary.fixed
model_random<-inlabru_model$summary.random
model_hyperparams <- inlabru_model$summary.hyperpar
The Deviance Information Criterion (DIC) value, which measures the goodness of model fit, can be examined as follows.
model_dic<-inlabru_model$dic$dic
You can obtain the model’s fitted values and compare them with the observed values as follows.
fitted_vals<-inlabru_model$summary.fitted.values[1:nrow(simdf),]
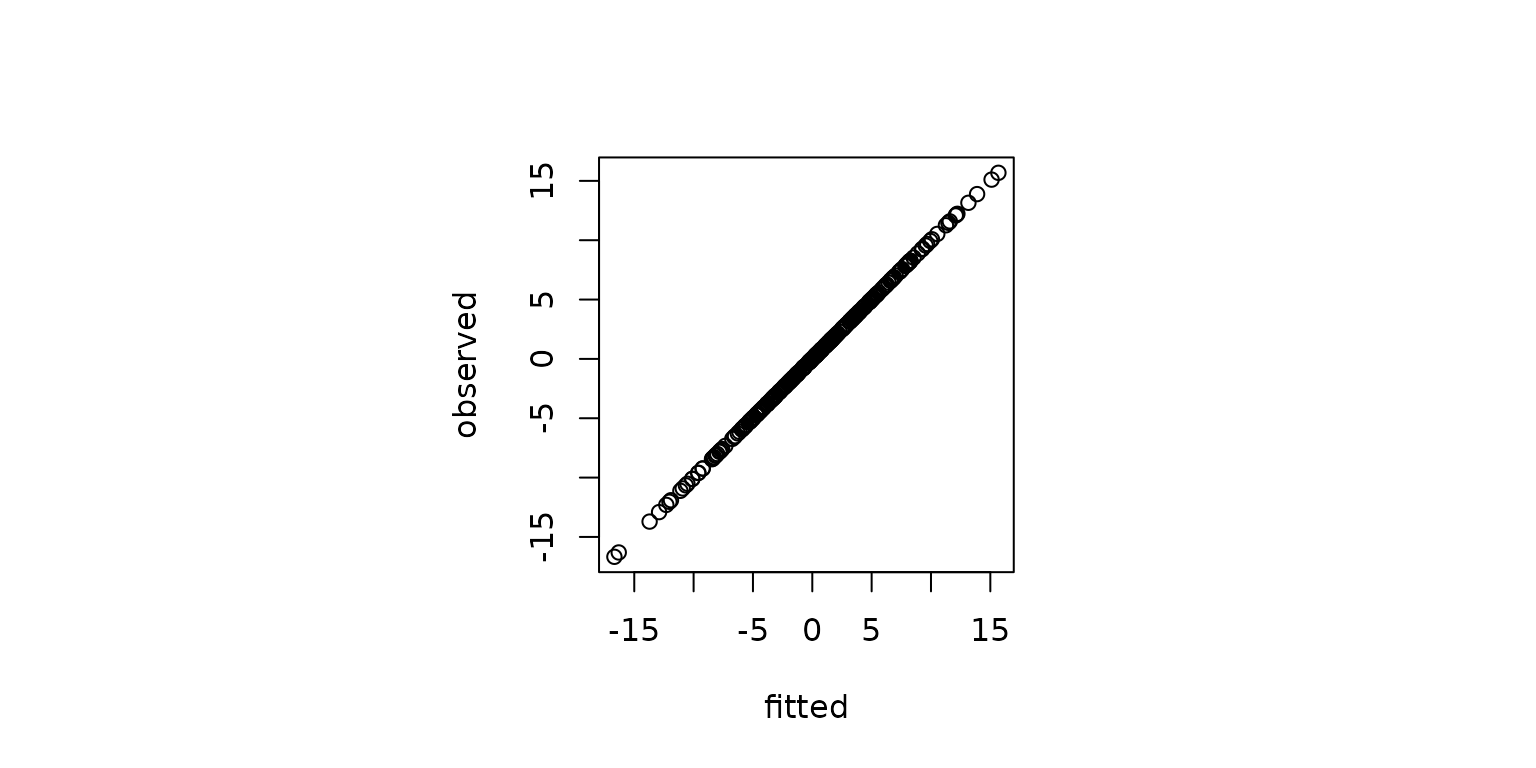
A plot of the fitted and observed values.
Exploring the functionality of the fdmr
Package
Next, we’ll demonstrate the key functionality offered by the
fdmr
package.
fdmr::plot_mesh
The fdmr::plot_mesh
function displays both the mesh and
the observation points overlaid on the mesh.
fdmr::plot_mesh(mesh = mesh, point_data = simdf@coords,
point_colour = "blue", cex = 0.8)
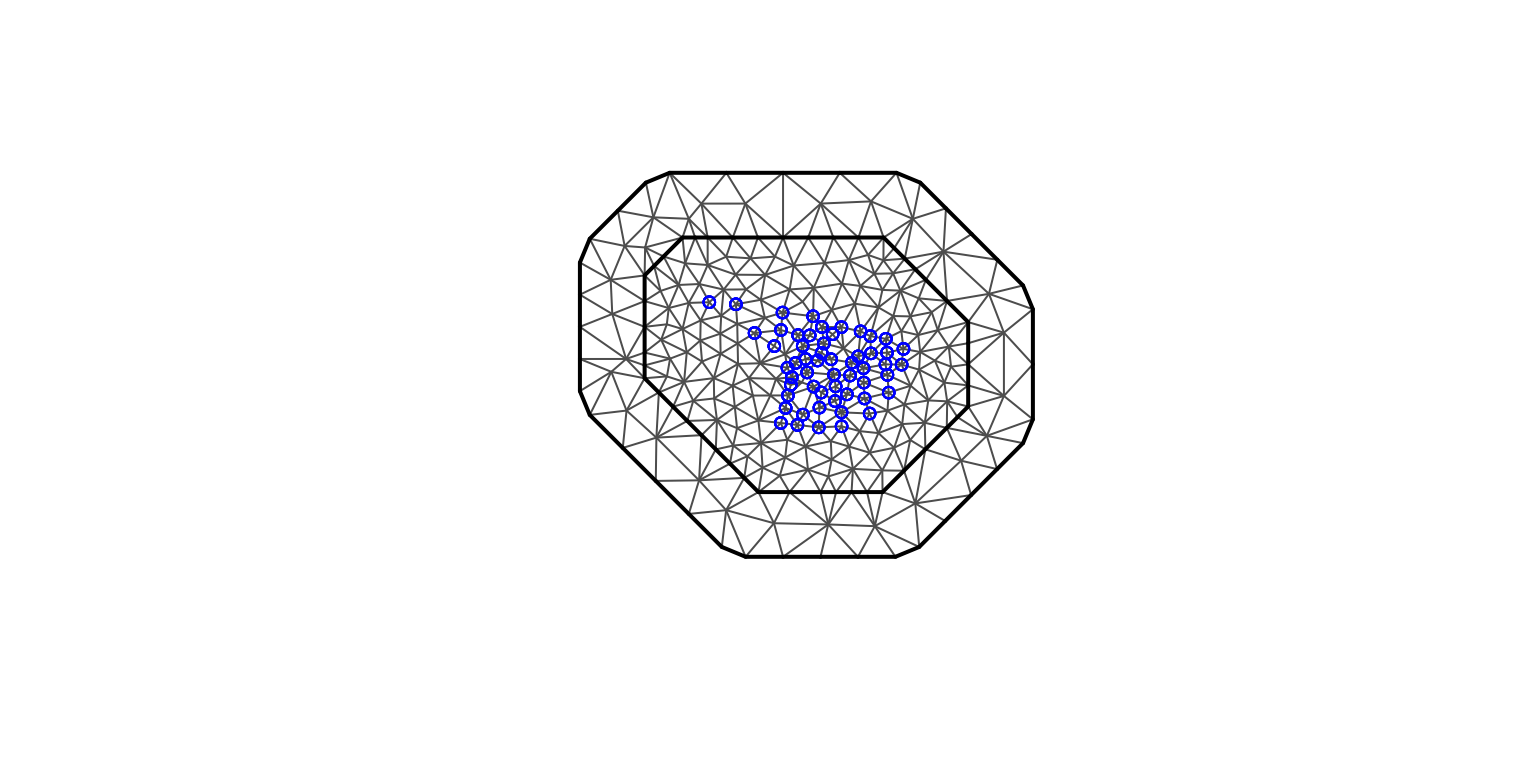
A plot of the mesh.
fdmr::mesh_builder
fdmr
provides an interactive mesh builder tool called
fdmr::mesh_builder
. It allows you to build a mesh from some
spatial data, modify its parameters and plot it and the data on an
interactive map. To build a mesh, we need to pass sp_data
to the mesh_builder
function.
fdmr::mesh_builder(spatial_data = sp_data)
The interactive map allows you to customise the initial parameters
such as max_edge
, offset
and
cutoff
to change the shape and size of the mesh. We also
provide a simple function to check if there are any errors with the
meshes created using the mesh builder tool. To use the mesh checking
functionality you must pass your measuremnet data to the
fdmr::mesh_builder
function. Specifically, create a mesh of
your design using the meshbuilder tool. When you’re finished, click the
“Check mesh” button on the user interface. This passes the created mesh
to the fdmr::mesh_checker
function and returns a list
containing any errors found with the mesh.
fdmr::mesh_builder(spatial_data = sp_data, obs_data = simdf)
Interactive priors Shiny app
fdmr
provides a Priors Shiny app which allows you to
interactively set and see the model fitting results of different priors.
You can launch this app by passing the spatial and observation data to
the fdmr::interactive_priors
function.
fdmr::interactive_priors(spatial_data = sp_data,
measurement_data = simdf,
mesh=mesh,
time_variable="time")
fdmr::plot_map
The fdmr::plot_map
function generates a map of the
region based on the provided SpatialPolygonDataFrame.
fdmr::plot_map(polygon_data = sp_data)
A map of the study region.